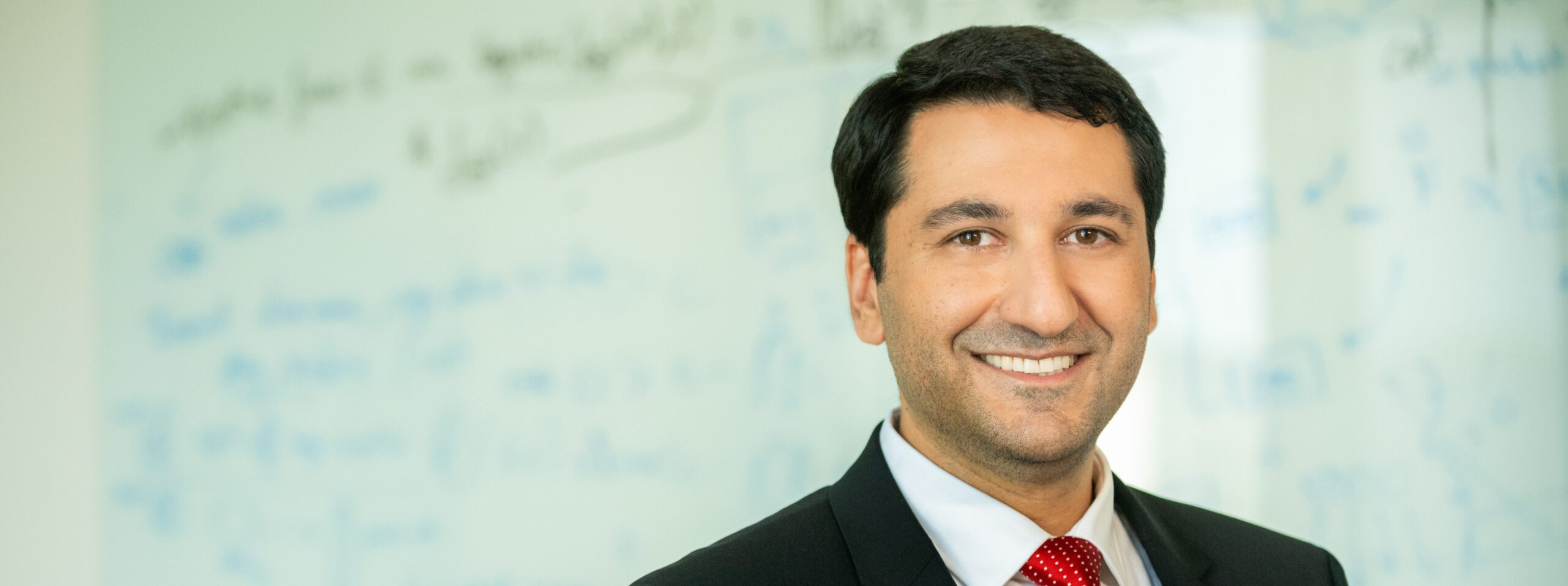
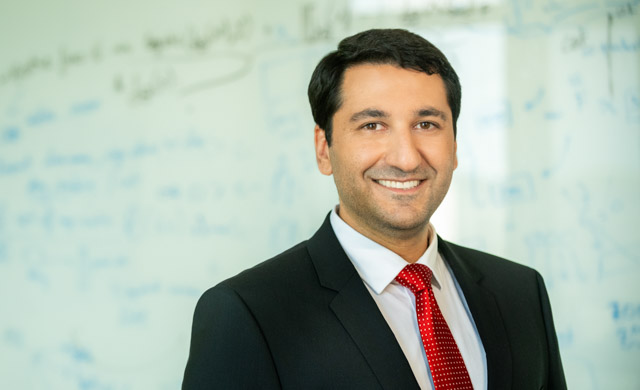
Ehsan Elhamifar
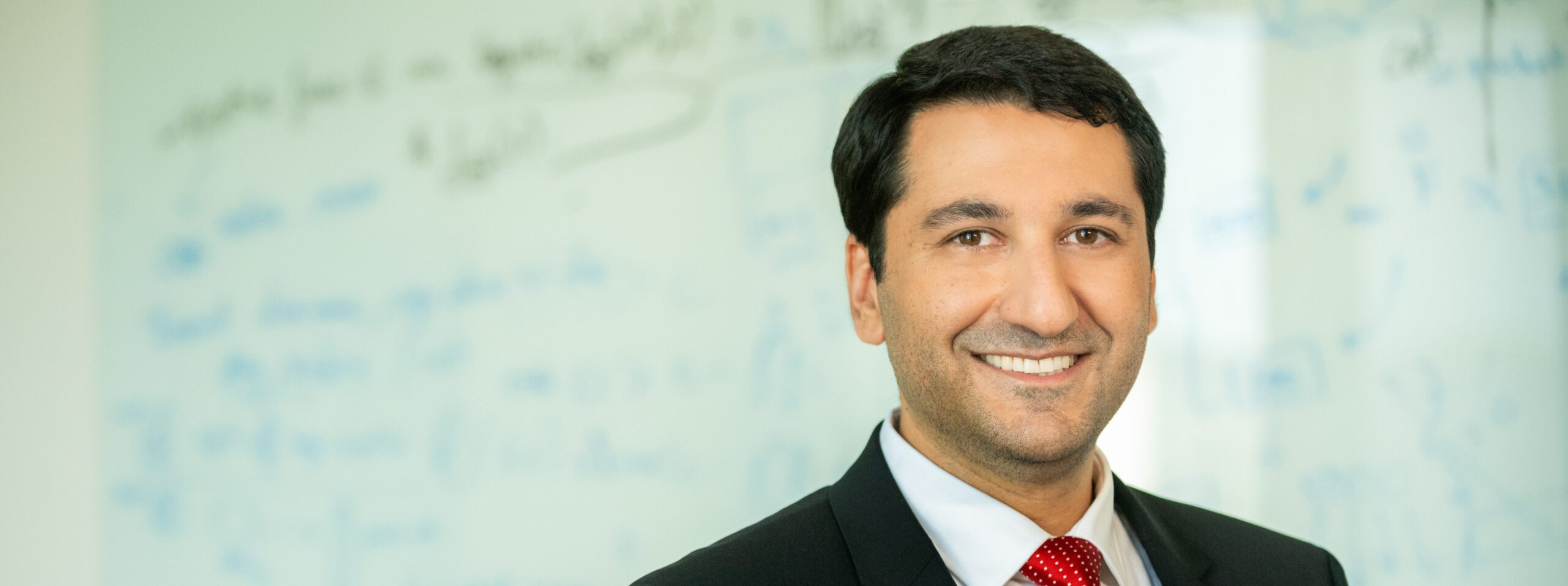
Associate Professor, Affiliate Faculty with the Department of Electrical and Computer Engineering
Boston
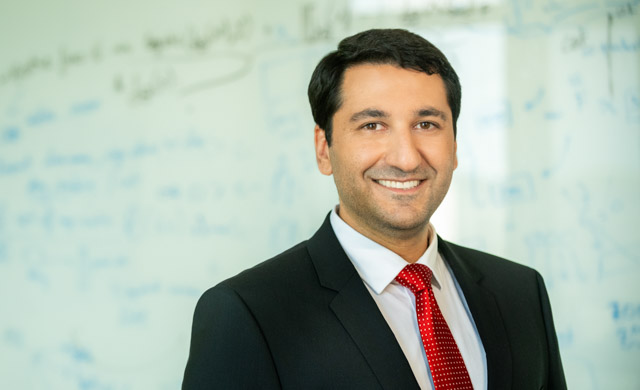
Ehsan Elhamifar
Associate Professor, Affiliate Faculty with the Department of Electrical and Computer Engineering
Boston
Associate Professor, Affiliate Faculty with the Department of Electrical and Computer Engineering
Boston
Associate Professor, Affiliate Faculty with the Department of Electrical and Computer Engineering
Boston
Associate Professor, Affiliate Faculty with the Department of Electrical and Computer Engineering
Boston
Associate Professor, Affiliate Faculty with the Department of Electrical and Computer Engineering
Boston
Ehsan Elhamifar is an associate professor in the Khoury College of Computer Sciences and director of the Mathematical, Computational and Applied Data Science Lab at Northeastern University. He is affiliated with Northeastern’s electrical and computer engineering department. Elhamifar is a recipient of the DARPA Young Faculty Award and the NSF CISE Career Research Initiation Initiative Award. Previously, he was a postdoctoral scholar in the electrical engineering and computer science department at University of California, Berkeley.
Elhamifar’s research areas are machine learning, computer vision, and optimization. He is interested in developing scalable, robust, and provable algorithms to address challenges of complex and massive high-dimensional data. He applies these tools in areas such as computer vision and robotics. Specifically, he uses tools from convex, non-convex, and submodular optimization; sparse and low-rank modeling; deep learning; high-dimensional statistics; and graphical models to develop algorithms and theory. He applies these discoveries to real-world challenges, including big data summarization, procedure learning from instructional data, large-scale recognition with small labeled data, and active learning for visual data.
Ehsan Elhamifar is an associate professor in the Khoury College of Computer Sciences and director of the Mathematical, Computational and Applied Data Science Lab at Northeastern University. He is affiliated with Northeastern’s electrical and computer engineering department. Elhamifar is a recipient of the DARPA Young Faculty Award and the NSF CISE Career Research Initiation Initiative Award. Previously, he was a postdoctoral scholar in the electrical engineering and computer science department at University of California, Berkeley.
Elhamifar’s research areas are machine learning, computer vision, and optimization. He is interested in developing scalable, robust, and provable algorithms to address challenges of complex and massive high-dimensional data. He applies these tools in areas such as computer vision and robotics. Specifically, he uses tools from convex, non-convex, and submodular optimization; sparse and low-rank modeling; deep learning; high-dimensional statistics; and graphical models to develop algorithms and theory. He applies these discoveries to real-world challenges, including big data summarization, procedure learning from instructional data, large-scale recognition with small labeled data, and active learning for visual data.