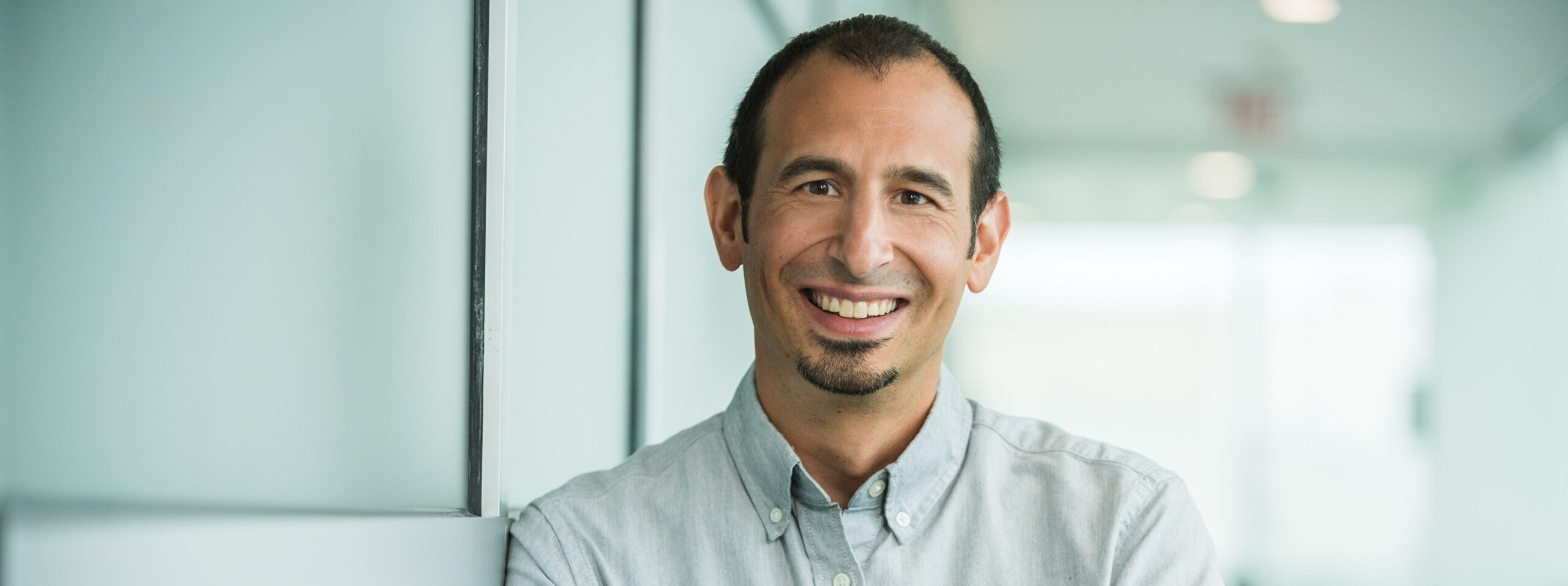
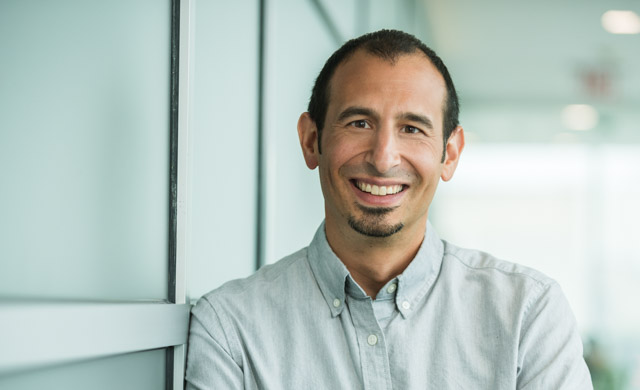
Christopher Amato
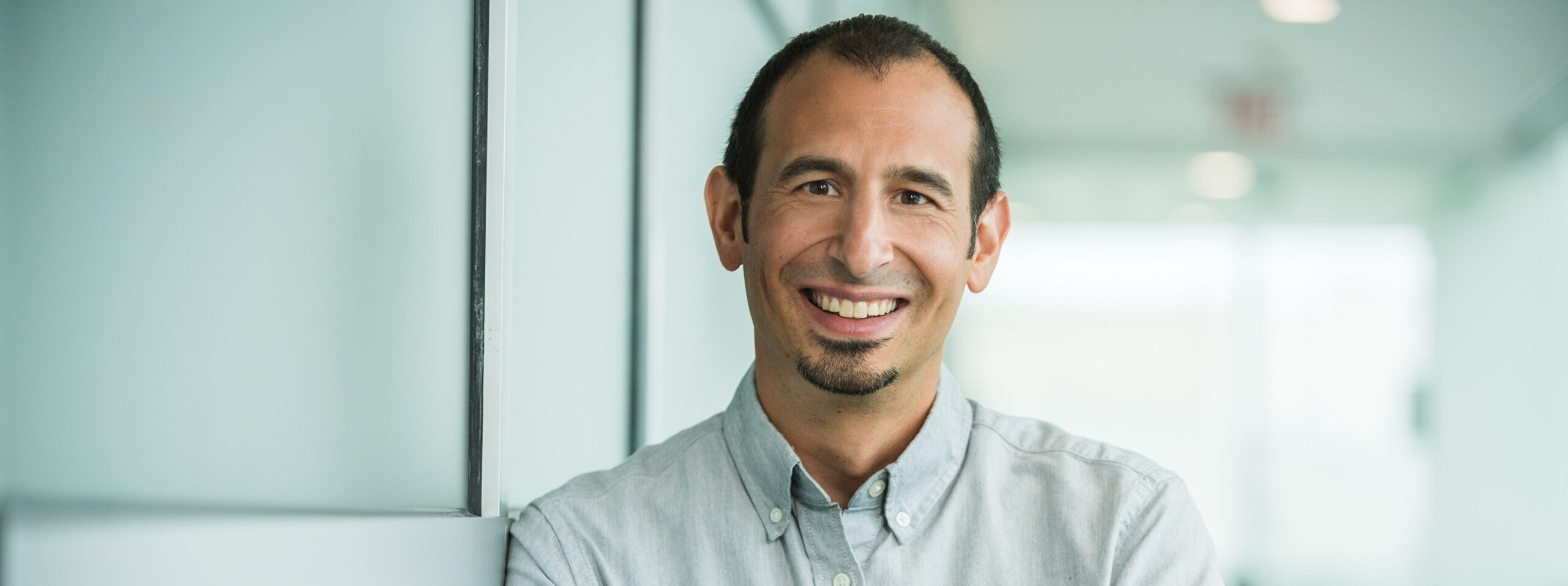
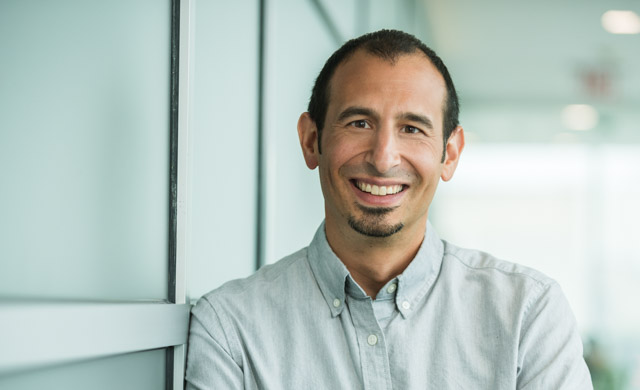
he/him/his
Christopher Amato is an associate professor in the Khoury College of Computer Sciences at Northeastern University. His research is at the intersection of artificial intelligence, machine learning and robotics. Amato currently heads the Lab for Learning and Planning in Robotics, where his team works on planning and reinforcement learning in partially observable and multi-agent/multi-robot systems.
Before joining Northeastern, he worked as a research scientist at Aptima Inc., a research scientist and postdoctoral fellow at MIT, and an assistant professor at the University of New Hampshire. Amato received his bachelor’s from Tufts University and his master’s and doctorate from the University of Massachusetts, Amherst.
Amato is widely published in leading artificial intelligence, machine learning, and robotics conferences. He is the recipient of a best paper prize at AAMAS-14 and was nominated for the best paper at RSS-15, AAAI-19, and AAMAS-21. Amato has also successfully co-organized several tutorials on multi-agent planning and learning and has co-authored a book on the subject.
he/him/his
Christopher Amato is an associate professor in the Khoury College of Computer Sciences at Northeastern University. His research is at the intersection of artificial intelligence, machine learning and robotics. Amato currently heads the Lab for Learning and Planning in Robotics, where his team works on planning and reinforcement learning in partially observable and multi-agent/multi-robot systems.
Before joining Northeastern, he worked as a research scientist at Aptima Inc., a research scientist and postdoctoral fellow at MIT, and an assistant professor at the University of New Hampshire. Amato received his bachelor’s from Tufts University and his master’s and doctorate from the University of Massachusetts, Amherst.
Amato is widely published in leading artificial intelligence, machine learning, and robotics conferences. He is the recipient of a best paper prize at AAMAS-14 and was nominated for the best paper at RSS-15, AAAI-19, and AAMAS-21. Amato has also successfully co-organized several tutorials on multi-agent planning and learning and has co-authored a book on the subject.